Predictive energy modelling is the defining characteristic of Passive House design in my view, if I were forced to choose only one thing. There is a wide range of modelling tools that can and are used in building design. In this month’s technical article, I offer some advice about which modelling tools to never use, which I recommend and those that our team reserves for very specific circumstances.
Firstly, use predicative not indicative models for design (I expand on this at the end). Unsurprisingly, among the predictive models, I recommend using PHPP modelling software, which is a monthly energy balance single zone model.
Zonal dynamic predictive models
There are tools that create very detailed zonal and/or dynamic models. Our team uses such software tools (and love them), but only occasionally and in quite specific circumstances. Why? In part because they are expensive to run. But it’s also a matter of precision and accuracy. These are two different things, although it’s very nice when they align.
Zonal and dynamic models are great fun. We can report to a client that the model predicts the temperature will be 21.34C in the kids’ bedroom on a typical spring afternoon—but if the walls were painted dark blue, it’ll reach 22.43C. I can drill down into the impact of rug colour or interior door open times! However, the amount of data to look at becomes overwhelming very quickly, even for a single design on a single fixed site. There needs to be a compelling reason to consider dynamic modeling.
While a full dynamic model gives very precise results, we can’t assume those results are more accurate. They very often aren’t, at least outside of academic research which generally has the budget to be very thorough. It takes a lot of time to review and set thousands of inputs (and the experience and building physics knowledge to readily identify which inputs to focus on) plus you need a QC check. Accuracy of results in large part rests on how much time and therefore money is available. As for running the model on default inputs? Yes it sure saves time but I can tell you from my own work that the default results are less accurate than a much simpler model that has been provided with relevant inputs. Out by 50% is not uncommon: we’re not talking small margins of error. To reiterate, accuracy is more important than precision if you have to choose between them.
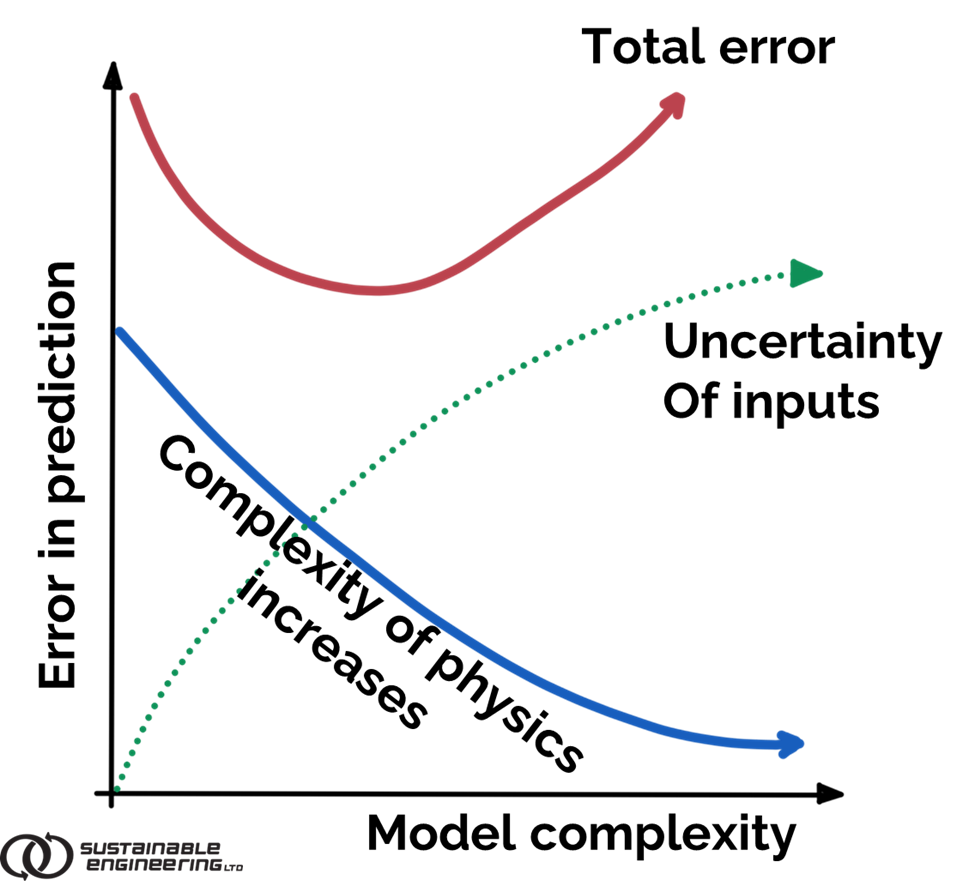
Potential error in performance prediction versus model complexity/level of detail. Simplified graph based on: Building Performance Simulation for Design and Operation,Edited Hensen, J., and Lamberts, R., Spon Press 2011, pg 11.
Also be aware that the evidence is that as models become more complex, the potential for error in results increases. That’s the basic takeaway from the above graph. What’s interesting is the U-shaped curve for total error. When the physics is really basic, the error rate is high, as you’d expect. As the physics get more complicated (moving right along the blue curve), the error rate drops. But at a certain point, the red line (the total error rate) starts to increase again. The model has become so complex that the uncertainty of inputs increases the error in prediction of the model. We want our modelling to fit into the sweet spot on the error curve. Pick the right tools for the job.
Key takeaways
- Don’t use complicated models to solve simple problems.
- Don’t rely on default inputs for complex models if you want accurate results.
- Don’t use NZBC calculation models for jobs that need predictive power.
Do not use indicative models
Lastly, my views on this are already on the record but once again for emphasis: do not waste your time using indicative models. We need predictive modelling, such as what PHPP provides. All useful models should predict the performance of the building in absolute terms. A Building Code compliance model is not predictive, that’s not its function. It exists to demonstrate compliance with the Building Code and on that basis excludes real building physics. If the results are then ill-advisedly used to compare the performance of different variables, the results are often misleading.
I’ll work through an example to illustrate the point. Take internally insulated concrete walls in a commercial building. The New Zealand Building Code calculation method permits counting from the top of floor to the underside of the finished ceiling, ignoring junctions. So the wall above the ceiling is uninsulated (maybe it’s R0.2). The floor slab edge is uninsulated. Corners, columns and window installs are uninsulated. Etc! The wall R-value is R2 in name but only because it ignores all of those other bits. The modelling would indicate thermal losses would halve if wall insulation were increased to R4. This is not true according to real world physics because almost all of the losses are from those parts of the wall-to-floor junction(s) that are excluded from the model.
The effects are so bad in general practice that mechanical engineers designing commercial HVAC systems won’t use the Building Code compliance model to size the heating and cooling system as they know the results would be totally wrong.